Introduction
Companies are rapidly adopting AI, changing how they work and innovate. A recent McKinsey & Company study reflects a significant increase in AI adoption by various business functions from 2021 to 2024. Marketing and sales, IT, and service development are leading the way. They’re using Generative AI to be more productive, efficient and cost effective. On the flip side, it also brings new challenges.
The risk of AI hallucinations can jeopardize the trust in this cutting-edge technology.
Have you ever been in this situation?
You asked Generative AI a straightforward question expecting a clear, reliable and factual answer. In return you received a very convincing answer. On second thought, you decided to fact check and found that the AI’s response was fabricated and misleading.
Sounds relatable?
This phenomenon is often referred to as “hallucinations” in AI. It is surprisingly common in customer facing companies and has ended in frustrated customers, lawsuits, reputation damage and financial loss.
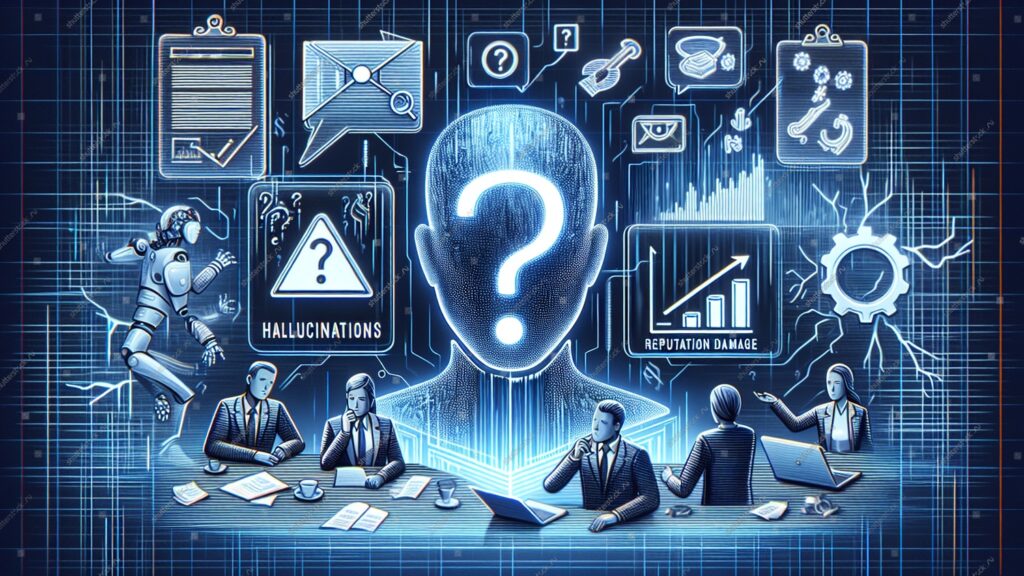
Often when we speak of hallucinations, we refer to a faulty perception wherein whatever vision or patterns are being observed are not real. Just like a mirage tricks the human eye, AI hallucinations stem from misinterpretations or overgeneralizations in the model’s training data. This can lead to nonsensical and inaccurate outcomes.
Let’s explore some real-world examples to get a better understanding.
Generative AI Hallucination Examples
1) The Air Canada Incident
The recent Air Canada incident is an example of how AI hallucinations can wreck havoc on a company’s reputation and finances.
The accounts of the incident are as follows:
- One passenger reported being misled by inaccurate information provided by an airline chatbot about bereavement fees, which went against official airline policy.
- The Tribunal in Canada’s small claims court sided with the passenger and awarded them $812.02 in damages and court costs. Incorrect answers to the chatbot were considered “Negligent Misrepresentation”.
- Christopher C. McCarthy, in his judgment emphasized that, while chatbots provide an interactive experience, they are ultimately part of Air Canada’s website. “It should be clear to Air Canada that it bears responsibility for all content on its website, whether from a blank page or a chatbot,” Rivers said.
2) Job applicants using Indirect Prompt Injections in their resumes
Nowadays a lot of the HR companies are screening resumes and documents using AI to escape a deluge of generated content.
But here is the catch: by taking advantage of AI’s hallucinating weakness, candidates sometimes use a technique to bypass the AI screening. This is called prompt injection. There are websites that allow users to inject invisible text into their PDF, fooling the AI language model into thinking the candidate as the perfect fit.
According to the Learn Prompting website, prompts hacking can be classified as:
- Prompt Injection:
- Direct Prompt Injection
- Indirect Prompt Injection
- Stored prompt Injection
- Prompt Leaking
- Jailbreaking
What Causes AI Hallucinations?
AI hallucinations often result from gaps in training data. Picture yourself trying to complete a jigsaw puzzle with pieces missing. This will leave you guessing the wrong things. When AI systems don’t get enough exposure to key information during training, they end up creating output that makes no sense at all.
Another big reason is flawed assumptions in the model and biased training data. If the AI is built on shaky logic or learns from biased examples, it can twist the results. It’s like someone who hears one side of a story. On top of this, there’s the issue of the model’s overconfidence. It gives wrong answers but sounds very sure of itself. This false certainty can trick people into thinking the AI’s made-up information is true at first. To build smarter, more dependable AI systems, we need to overcome these flaws.
The Impact of Neglecting AI in Your Organization
With a huge adoption rate of AI, organizations can no longer afford to sit idle and neglect the AI systems. This can lead to costly consequences like:
- Data Breaches
- Financial Losses
- Reputation Damage
- Regulatory Penalties
- Operational Disruption
- Intellectual Property Theft
- Ethical and Legal Issues
Attackers are harnessing the power of large language models (LLMs) to create highly convincing phishing emails, posing a significant threat to organizations. To counteract this, businesses relying on LLMs must exercise extreme caution, especially when using AI-generated outputs for critical decisions. Preemptive measures are essential to mitigate the risks of AI hallucinations, instances where AI may produce inaccurate or misleading information.
By staying vigilant and adopting robust validation processes, organizations can protect themselves against these evolving threats.
How to Prevent AI Hallucinations with Responsible AI
The antidote to AI hallucinations and to malpractices surrounding AI are:
- Focus on High-Quality Training Data: Use varied, correct, and unbiased datasets that show real-world situations, including unusual cases. Often update and grow datasets to help AI adjust to new facts and cut down on mistakes.
- Stick to Verified Sources: Use training data from trusted, expert sources to lower the chance of learning from wrong or uncertain information. A chosen dataset ensures correctness and cuts down on made-up responses.
- Use Data Templates: Implement structured templates to guide AI responses making sure they stay consistent and correct. Templates work well for standard tasks like making reports keeping outputs in line with wanted formats.
- Privacy: Protect personal data in AI systems and handle it in line with data protection laws.
- Ethics: AI development and use should be fair, and respect human rights, addressing moral issues.
- Security: AI services need protection from cyber threats to keep them intact, available, and confidential.
- Risks: AI technologies come with possible downsides, like technical problems and ethical issues, which need to be found, checked, and reduced.
- Bias and Fairness: AI systems should not make current biases worse or create new ones aiming to give fair results to all users.
- Transparency: Users and stakeholders should be able to understand and explain AI systems, which helps build trust and use these tools well.
- Accountability: Setting up clear responsibility chains for AI system results and making sure there are ways to tackle any harmful effects.
- Human Fact Checking: No matter how much AI advances, stick to the human nature of fact checking, validating and then making decisions.
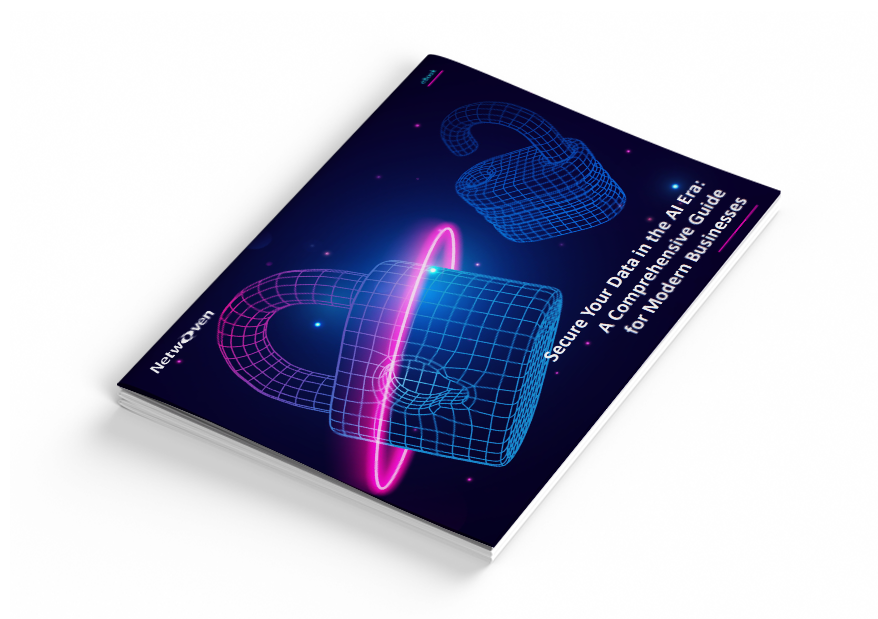
Ebook: Secure Your Data in the AI Era
The rapid evolution of AI requires organizations to update data security protocols to protect sensitive information. This eBook provides practical guidelines to help strengthen data security in the AI era.
Get the eBookConclusion
In the face of increasing complexity, securing AI systems has become an all-time priority for organizations. The ever-increasing pace of advancement in AI, coupled with geographically distributed teams and tools, poses significant challenges to security teams. They often work within a low-visibility realm of AI-driven systems and thus have a harder job in detecting vulnerabilities and minimizing risks. Moreover, the changing landscape of regulation and ethics requires organizations to build robust governance frameworks that conform to emerging standards while keeping pace with the unique threats that AI poses in offensive and defensive security.
With a holistic approach that includes specialized knowledge, innovative tools, and enhanced governance strategies, Netwoven enables security teams to protect their AI investments. By partnering with Netwoven, organizations can confidently adopt AI technologies, ensuring that their systems are resilient against cyber threats and compliant with evolving regulatory demands, paving the way for secure and innovative growth. For any queries, please reach out to us.