What is Data Observability
Data observability empowers organizations to grasp the vitality of their data systems, utilizing DevOps Observability principles to eradicate data downtime. Through automated monitoring, alerting, and triaging, it identifies and assesses data quality and discoverability concerns, fostering robust data pipelines, enhancing team productivity, and ensuring satisfaction among data consumers.
Five Pillars of Data Observability
Data Freshness
Assessing the timeliness and update frequency of your data tables is crucial for informed decision-making. Outdated data equals wasted time and money.
Data Distribution
Understanding the range of possible values in your data provides insights into its reliability. Knowing what to expect from your data helps establish trustworthiness.
Data Volume
The completeness of your data tables reflects the health of your data sources. Sudden changes in volume alert you to potential issues.
Data Schema
Changes in data organization can indicate data integrity issues. Tracking schema modifications and their timing is key to maintaining a healthy data ecosystem.
Data Lineage
Pinpointing where data breaks occur is essential. Lineage reveals the upstream sources and downstream users affected, along with metadata essential for governance and consistency.
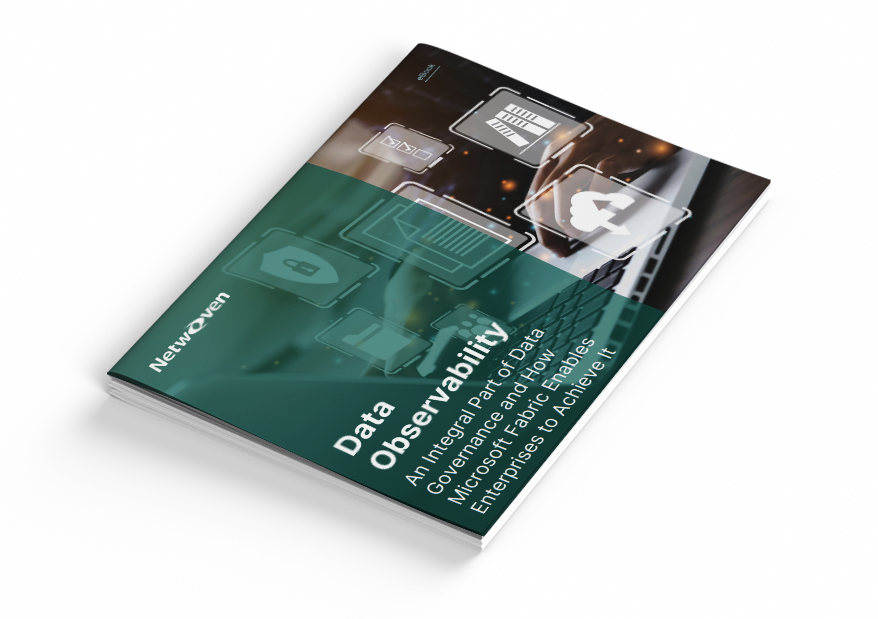
Data Observability with Microsoft Fabric
Data observability is the ability of an organization to have broad visibility of its data landscape and multilayer data dependencies. It helps bridge the gaps in data governance, ensuring a well-rounded, comprehensive, and contextual approach to resolving bottlenecks and driving results.
Get the eBookKey Components of Data Observability
Rapid Integration
Does it seamlessly integrate with your existing infrastructure without the need for pipeline modifications, coding, or specific programming languages? A swift and smooth connection ensures quicker benefits and enhanced testing coverage without substantial investments.
Security-Centric Architecture
Does it monitor data in its current storage location without necessitating data extraction? Such a solution scales across your data platform, ensuring cost-effectiveness and compliance with stringent security standards.
Simplified Setup
Does it require minimal configuration and virtually no threshold adjustments to get started? An effective data observability platform employs machine learning models to adapt to your environment and data automatically. By utilizing anomaly detection techniques, it reduces false positives by considering the broader context of your data, minimizing the need for extensive rule configuration and maintenance. Moreover, it allows you to define custom rules for critical pipelines directly within your CI/CD workflow, offering both simplicity and flexibility.
Webinar: Data Observability with Microsoft Fabric. Watch Now.
Core fundamental foundations
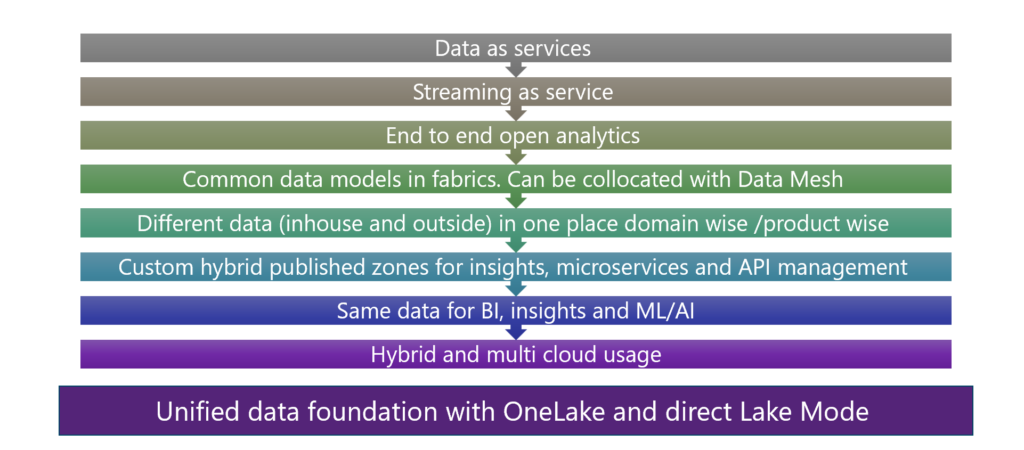
Conclusion
In summary, implementing data observability is crucial for organizations aiming to maximize the value and reliability of their data systems. By embracing the five pillars of data observability—data freshness, distribution, volume, schema, and lineage—and utilizing key components such as rapid integration, security-centric architecture, and simplified setup, organizations can achieve greater insights, productivity, and satisfaction among data consumers.
Microsoft Fabric, specifically Onelake, serves as a robust supporter of the data observability framework, offering core elements that benefit customers immensely. Its emphasis on time-to-value, security-first architecture, and minimal configuration aligns perfectly with the needs of modern organizations. By seamlessly integrating with existing stacks, ensuring data security at rest, and requiring minimal setup and configuration, Microsoft Fabric simplifies the path to achieving comprehensive data observability. With its inclusive and integrated approach, facilitated by one-tenant deployment, Microsoft Fabric emerges as a reliable partner in fostering a data-driven culture within organizations, ultimately driving success in today’s data-centric landscape.